The hot topic in the world of big data analytics right now is validation.
What exactly is it, and why is it so important?
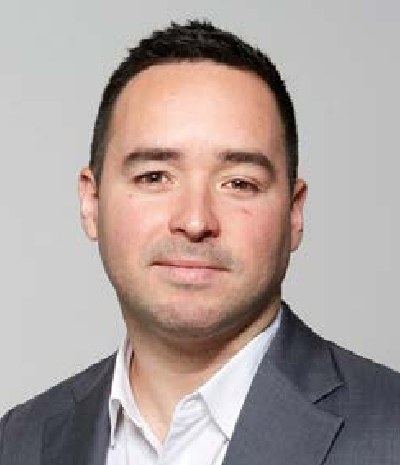
Daniel Porragas, Affil.AIRAH
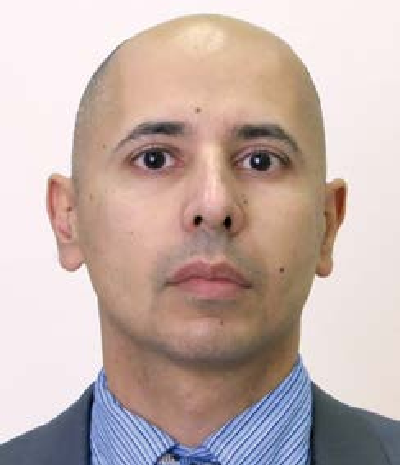
Evren Korular, M.AIRAH
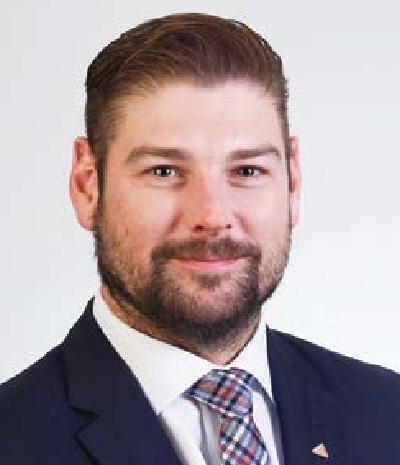
Rob Huntington, M.AIRAH

Roshan Gill, M.AIRAH
Ecolibrium: What is “validation” in a big data context?
Daniel Porragas: It refers to the combination of processes, practices, techniques, or mechanisms (manual or automated) that ensure the data generated by various sensors, systems, and devices within a building is accurate, reliable, consistent, and useful for the intended purposes. This data could include temperature readings, energy consumption levels, occupancy numbers, and more.
Data validation techniques might include checking for and correcting errors, removing, or addressing outliers, ensuring that the data meets certain rules or standards, and more. Some of the techniques used could be statistical methods, machine learning algorithms, rule-based checks, or even manual commissioning processes such as an energy meter face reading versus the energy management system head-end graphics.
Evren Korular: Building data validation ensures that the terms and properties defined in an ontology are used correctly within a specific instance, while also adhering to the formal model without any logical conflicts. In simpler terms, validation verifies the accuracy of metadata models.
Rob Huntington: Where there is a specific requirement for how data is to be structured – that is, a methodology for semantic data modelling – such as Brick or Haystack – or even something as simple as a naming convention – validation confirms that the structure has been adhered to. In the case of semantic data modelling, it is confirmation that the data model exists.
Roshan Gill: Validation relates to authenticating a convention or structure that has been applied to a dataset based on a standard of specification. As the industry is continuing to innovate in this space, it is paramount to be able to validate specified implementations against a structure, as well as substantiating new ideas for implementation methods in a collaborative environment. There are parallels that could be drawn with language and syntax, which evolve over time, and are often open to interpretation and subjective methods of expression.
Why do we need validation, and what value does it have for building owners, service providers and consumers (e.g., facility managers reporting on GRESB)?
DP: Without data validation, the data used in smart building systems could be inaccurate or inconsistent, leading to suboptimal performance, higher costs, and potential safety issues. For example, if a sensor is malfunctioning and providing incorrect temperature readings, it could cause the HVAC system to over- or under-condition the building, resulting in wasted energy and uncomfortable conditions for occupants. Therefore, data validation is a crucial aspect of managing and operating smart buildings effectively.
EK: The goal of validation is a consistent, machine-readable and verifiable digital representation of the building that supports data-driven applications. The solution consists of three main aspects: describing and contextualising building data, expressing data requirements for applications, and deploying building apps at scale.
For building owners, it can reduce initial costs as well as operating costs by minimising expenses associated with point mapping, commissioning, application installation, and configuration. Moreover, it will provide the capability to validate the accuracy of system integrators’ work.
For service providers, there are benefits in interoperability of building data by various applications, as well as easy access to information throughout the design, installation, and commissioning phases. Additionally, deployment will promote the standardisation of applications and enable the adoption of vendor-agnostic solutions.
For consumers, capabilities include analytics, dashboards, asset management, and advanced controls, offering enhanced functionalities. Furthermore, validation facilitates easier adoption and seamless upgrading of applications for continuous usage and improved functionalities.
RH: For building owners, validation unlocks the benefits of big data at scale. Particularly at a portfolio level, there has to be a standard between equipment, systems and buildings to allow new technology without costly deployment costs, which often require a data structure to be built within a third-party product or application, i.e., building analytics.
For service providers, in the first instance it would be about de-risking a project. For too long, despite things like tagging being hard-specced, it is rarely delivered due to the lack of validation tools – and perhaps a lack of use cases for the structured data. As clients become more aware of the importance of structuring their data, it will become more obvious when the data structure has not been adhered to.
In addition, it is not uncommon for a vendor to be awarded a contract when their product does not support the nominated schema! There are secondary benefits with relation to engineering efficiencies, such as tag-based graphics, which could also benefit the providers.
For consumers, validation offers the ability to automate tasks such as data collection, analysis and visualisation for reporting purposes, hypothetically at the click of a button. It also offers the ability to rapidly roll out and trial new and emerging technology without the cost of deployment.
RG: Over the life-cycle of a building, the owners save on expenditure when implementing applications on their buildings that are consumers of the data, by reducing implementation costs.
This assists with unlocking the value of data that all buildings have, as well as future-proofing to allow a wider spread of applications post-development or upgrade to a building system.
Service providers perform an integral role in the effective implementation of structured data in buildings. Because there are a variety of options for implementing an effective structure, their role in this needs to be cooperative. The value to them is being able to afford more sophistication and value in their service, as well as driving the industry forward.
And it allows consumers to derive additional insights into building performance by effective correlation of complex data, as well as implementing complex control strategies in relation to intelligent demand performance, and participation in energy markets.
Dive into the data
What are the technical, commercial and people challenges?
DP: Comprehensive data-validation strategies rely on a combination of detailed design, clear requirements, and engineering of the desired outcomes using one or multiple tools or solutions. Tight coordination between system designers, solution providers and building operators is required to achieve this, as there is no single system that can perform all these techniques out of the box. As with cyber-security and IT policies, data-validation outcomes may form part of a wider data policy that is constantly evolving and improving. This can come from either solution providers or building owners and operators.
EK: In terms of technical challenges, gaining a comprehensive understanding of both OT and IT applications and their integration is vital. Validation tools play a crucial role in ensuring accuracy by providing binary reports that indicate the validity of equipment information.
On the commercial front, abstraction is key to interoperability – we need scalability. Creation of a purposeful metadata database is crucial, prioritising the inclusion of actively utilised information while avoiding unnecessary data. The Building Metadata Ontology Interoperability Framework (BuildingMOTIF) provides a comprehensive toolset for creating, storing, visualising, and validating building metadata. This framework effectively bridges the gap between theory and practice.
When it comes to people, by understanding the functional requirements and use cases, the focus can be placed on prioritising the creation of a database with relevant and meaningful metadata. Implementing a feedback mechanism becomes crucial to validate that the human input contributes to a model capable of effectively supporting the desired software.
RH: In terms of technical challenges, there is still a lack of understanding about the difference between semantic data models and naming conventions. It is very common for a metadata schema to be specified as a naming convention, which is not the intent. There is still an education piece that needs to take place to ensure people specifying understand what they are asking for and how to validate it.
Commercially, there is perhaps a perception that conforming to a standard will cost more; however, that is debateable. If the model is not applied at the edge, someone else will have to apply the model at the application layer, which will also have an associated cost. And the model then resides within that application, so if you want to change solution providers or trial other technology, the model will have to be built again by the next provider.
In addition, the closer you are to the system, the more likely it is that the model will be accurate. For an application provider to apply a model to a system they are not familiar with takes time and requires assumptions, particularly when it comes to contextual tagging.
But the biggest challenge is probably people. As an industry we like to keep doing things the way they have always been done. There is probably a level of protectionism going on, as applications such as building analytics have been viewed as “policing” the BMS service and maintenance. There has always been a culture of clients being held to ransom by proprietary systems, and the inability to conform to a client’s requirements around data structure is the modern-day version of this
RG: Technical challenges include the wide array of semantics and ontologies, which are subjective in implementation, and proprietary technology alignment. A commercial challenge is the increased costs during initial development, although these are outweighed by future retrospective implementation.
When it comes to people, the challenge is mindsets – achieving a consensus while maintaining the interests of multiple stakeholders.
Checks and balances
NDY senior project consultant Daniel Porragas, Affil.AIRAH, shares some common data validation techniques.
Range checks: This involves setting an acceptable range of values for a dataset. For instance, for a temperature sensor in a building, any values outside a plausible range (such as -50°C or 100°C) can be identified as likely errors.
Consistency checks: This ensures that the data doesn’t contradict other data. For instance, if two sensors in the same area of a building give very different temperature readings, there may be an inconsistency.
Completeness checks: This validates that no essential data is missing. If a sensor is supposed to provide data every 15 minutes and there is a two-hour gap in the data, a completeness check would flag this.
Format checks: This checks if the data is in the correct format. For example, dates should be in a specific format (e.g., MM-DD-YYYY), and numerical data should not contain letters.
Cross-checks: This compares data from different sources. For example, data from a light sensor could be cross-checked with the time of day and expected sunlight levels.
Data profiling: This involves statistical analysis and assessment of data for consistency and uniqueness.
Machine learning advanced methods: These can use machine learning or AI to identify anomalies or patterns that may signify data issues.
What happens if industry does not continue to innovate in this space?
DP: Progress in this field can trigger a beneficial cycle. As data validation techniques become more refined, innovative companies, industry organisations, and academic institutions can devote their efforts to analysing, modelling, and testing new data-driven technologies. This advancement reduces the need to constantly mitigate issues caused by data mistrust or resources spent in rectification, which are common when working with unreliable data. Consequently, these stakeholders can focus on driving forward meaningful innovations, promoting both efficiency and growth in the industry.
EK: Ontologies are essential for enabling seamless data interoperability and integration across diverse systems and domains. Their absence could impede the smooth exchange and integration of data, imposing limitations on collaboration and slowing progress.
To ensure the continuous development and enhancement of ontologies, it is crucial to foster collaborative efforts, initiate standardisation initiatives, and actively involve industry experts and researchers.
We consider AIRAH’s Big Bata STG to be a platform for nurturing individuals and fostering innovative ideas that can educate the industry about the advantages of data harmonisation.
RH: If we don’t, someone else will. There are already multiple open-source initiatives that are aiming to democratise data and make it more easily accessible and understandable. Those who do find ways to structure their data in accordance with emerging demands will succeed and will be well positioned to create meaningful partnerships with their clients
RG: Change and innovation in technology is always slowed by a general comfort level in the status quo. To reference a quote commonly attributed to Henry Ford: “If I had asked people what they wanted, they would have said faster horses.” The users of a technology should be engaged and consulted.
At the end of the day, they will be part of the solution. If industry does not continue to drive innovation in this space, the plethora of implementations and an effective narrowing of the consensus could be delayed, and cost more in the long run
Change and innovation in technology is always slowed by a general comfort level in the status quo.